报告题目:Reverse Attack: Practical Black-box Attack on Collaborative Recommendation
报告人:美国特拉华大学 袁旭 教授(NSF Career Award获得者)
主持人:姜楠 教授
时间:2023年12月26日(星期二)15:00-16:30
地点:威澳门尼斯人31188校友报告厅
报告人简介:
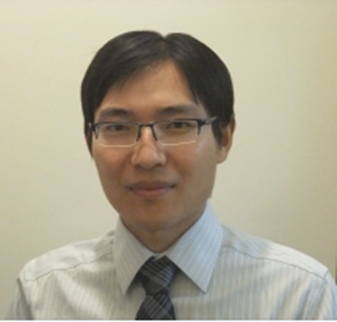
Dr. Xu Yuan is an Associate Professor in Department of ComputerInformation Science at University of Delaware. He received the B.S. degreethe College of Information Technology, Nankai University, China, in 2009,the Ph.D. degreeElectricalComputer Engineering, Virginia Tech, in 2016.2016 to 2017, he was a Postdoctoral Fellow of ElectricalComputer Engineering with the University of Toronto, Toronto, ON, Canada. His research interests focus on security, privacy, machine learning theory, AI applications,wireless networks. He has published more than 80 articles, including the top conferences/journals, such as ACM CCS, USENIX Security, IEEE INFOCOM, AAAI, IJCAI, KDD, ICDM, ICCV, IEEE ToN, IEEE TMC, IEEE JSAC, IEEE TPDS, etc. He was a receipt of NSF CRII award 2020, and the recipient of NSF CRII AwardNSF Career Award. He is currently serving as the Associate Editor of IEEE Transactions on Mobile ComputingIEEE Internet of Things.
报告摘要:
Collaborative filtering (CF) recommender systems have been widely deployed in various social websites (e.g., Amazon, Ebay, NetEase Music, etc), promoting productsservices to the users of interest. Meanwhile, extensive efforts have attempted at poisoning attacks to CF recommender systems for distorting the recommend results to reap commercialpersonal gains stealthily. However, all existing attacks belong to white-box attacks, which are impractical when applied to the real setting on online social websites. This talk will present a novelpractical black-box attack solution toward the CF recommender systems without knowing involved specific algorithms nor historical social data information a priori. Instead of directly attacking the unknown recommender systems, we perform certain operations on the social websites to collect a set of sampling data for use in constructing a surrogate model for deeply learning the inherent recommendation patterns. This surrogate model can estimate the item proximities, learned by the recommender systems. By attacking the surrogate model, the corresponding solutions (for availabilitytarget attacks) can be directly migrated to attack the original recommender systems. Our solutions have been demonstrated to be effective to item-based, matrix-factorization-based, neural network-based,graph structure-based CF recommendation systems.
欢迎大家参加!